Mario De Florio
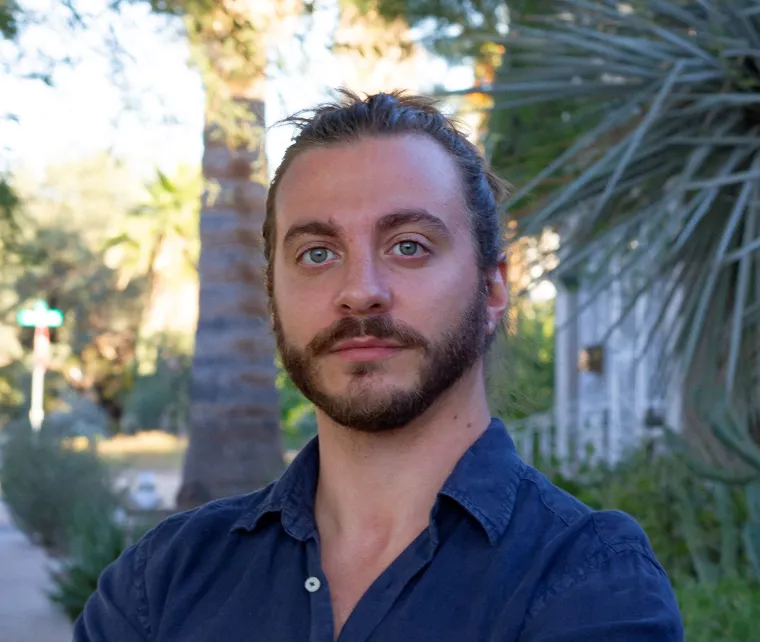
1127 E. James E. Rogers Way, Room 306, Tucson, AZ 85721
Documents
Mario De Florio was born in Foggia, Italy. He earned a bachelor's degree in Energy Engineering from the Alma Mater Studiorum - University of Bologna in July 2016 and a Master's degree in Energy and Nuclear Engineering in 2019 from the same University. He spent a semester abroad in Madrid, Spain, studying in the Nuclear Fusion field at Universidad Carlos III de Madrid and Universidad Complutense de Madrid, in 2018. He developed his Master's thesis work at The University of Arizona in 2019. From January 2020, Mario started the Ph.D. in Systems and Industrial Engineering at The University of Arizona, pursued in Fall 2022. Since Spring 2019, he has been developing a software for Binary Asteroid Systems characterization via light-curves inversion. During 2021 he has been developing models for meteorites parameters estimation by using Hapke models and Machine Learning. His main research interest is the development and application of Physics-Informed Neural Networks for various fields of science such as radiative transfer, rarefied-gas dynamics, nuclear reactor dynamics, chemical kinetics, and epidemiology.
He’s currently a Postdoctoral Research Associate at Brown University, Division of Applied Mathematics.
Degrees
- PhD, Systems and Industrial Engineering, The University of Arizona (2022)
- MSc, Energy and Nuclear Engineering, University of Bologna (2019)
- BSc, Energy Engineering, University of Bologna (2016)
Publications
Journal Articles
- Laghi, L., Schiassi, E., De Florio, M., Furfaro, R., and Mostacci, D., (2023) Physics-Informed Neural Networks for 1-D Steady-State Diffusion-Advection-Reaction Equations, Nuclear Science and Engineering, DOI: 10.1080/00295639.2022.2160604
- De Florio, M., Schiassi, E., and Furfaro, R., Physics-informed neural networks and functional interpolation for stiff chemical kinetics, Chaos 32, 063107 (2022). DOI: https://doi.org/10.1063/5.0086649
- De Florio, M., Schiassi, E., Ganapol, B.D. et al. Physics-Informed Neural Networks for rarefied-gas dynamics: Poiseuille flow in the BGK approximation. Z. Angew. Math. Phys. 73, 126 (2022). DOI: https://doi.org/10.1007/s00033-022-01767-z
- Schiassi, E., De Florio, M., Ganapol, B.D., Picca, P. and Furfaro, R., 2021. Physics-informed neural networks for the point kinetics equations for nuclear reactor dynamics. Annals of Nuclear Energy, p. 108833. DOI: https://doi.org/10.1016/j.anucene.2021.108833
- De Florio, M., Schiassi, E., D’Ambrosio, A., Mortari, D. and Furfaro, R., 2021. Theory of Functional Connections Applied to Linear ODEs Subject to Integral Constraints and Linear Ordinary Integro-Differential Equations. Mathematics, 26(3), p.65. DOI: https://doi.org/10.3390/mca26030065
- Schiassi, E., De Florio, M., D’Ambrosio, A., Mortari, D. and Furfaro, R., 2021. Physics-Informed Neural Networks and Functional Interpolation for Data-Driven Parameters Discovery of Epidemiological Compartmental Models. Mathematics, 9(17), p.2069. DOI: https://doi.org/10.3390/math9172069
- E. Schiassi, R. Furfaro, C. Leake, M. De Florio, H. Johnston, D. Mortari, Extreme Theory of Functional Connections: A Fast Physics-Informed Neural Network Method for Solving Ordinary and Partial Differential Equations, Neurocomputing (2021). DOI: https://doi.org/10.1016/j.neucom.2021.06.015
- De Florio, M., Schiassi, E., Ganapol, B.D., Furfaro, R., "Physics-informed neural networks for rarefied-gas dynamics: Thermal creep flow in the Bhatnagar–Gross–Krook approximation", Physics of Fluids 33, 047110 (2021). DOI: https://doi.org/10.1063/5.0046181
- De Florio, M., Schiassi, E., Furfaro, R., Ganapol, B.D., Mostacci, D. (2020). Solutions of Chandrasekhar’s Basic Problem in Radiative Transfer via Theory of Functional Connections. Journal of Quantitative Spectroscopy & Radiative Transfer. p.107384. DOI: https://doi.org/10.1016/j.jqsrt.2020.107384
Conference Papers
- Schiassi, E., D'Ambrosio, A., Johnston, H., De Florio, M., Furfaro, R., Curti, F., Mortari, D., Physics-Informed Extreme Theory of Functional Connections Applied to Optimal Orbit Transfer. AAS 20-524, AAS/AIAA Astrodynamics Specialist Conference, Lake Tahoe, CA, August 9-13, 2020. PDF
Preprints
- Schiassi, E., D'Ambrosio, A., De Florio, M., Furfaro, R., Curti, F. (2020). Physics-Informed Extreme Theory of Functional Connections Applied to Data-Driven Parameters Discovery of Epidemiological Compartmental Models. arXiv:2008.05554v1. PDF
- Schiassi, E., Leake, C., De Florio, M., Johnston, H., Furfaro, R., & Mortari, D. (2020). Extreme Theory of Functional Connections: A Physics-Informed Neural Network Method for Solving Parametric Differential Equations. arXiv :2005.10632. PDF
Conference Presentations
- 1st World Online Conference on Theory of Functional Connections, 22 May 2020. Physics-Informed Solutions for Rarefied Gas Dynamics Problems via Theory of Functional Connections. Presentation PDF
- 26th International Conference on Transport Theory, Paris (France), 22-27 September 2019. Accurate Solutions of the Radiative Transfer Problem via Theory of Connections. Conference Link, Presentation PDF
Conference Posters
- Los Alamos - Arizona days, Online Workshop, 18-19 May 2020. Physics-Informed Solutions of Radiative Transfer Problems. Poster PDF