Enrico Schiassi
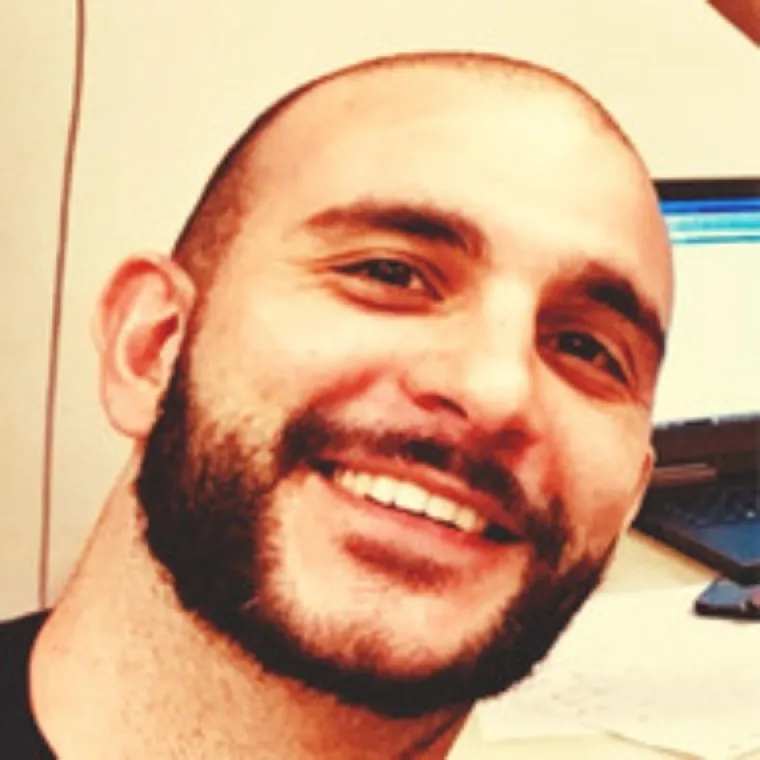
1127 E. James E. Rogers Way, Room 306, Tucson, AZ 85721
Links
Enrico Schiassi was born in Bologna, Italy. He has earned his bachelor's and master's degrees at the Alma Mater Studiorum University of Bologna in Energy Engineering, and Energy and Nuclear Engineering respectively. He developed his master thesis in spring 2016 within a collaboration between the University of Bologna and The University of Arizona, where he pursued his Ph.D in System Engineering in fall 2022. From fall 2017 until summer 2019 he worked within the NASA High Mountain Asia project (HiMAT) developing a software for glacier lakes characterization via satellite images. Since Spring 2019, he has been developing a software for Asteroid Binary Systems characterization via light-curves inversion. Since the summer of 2019, he is working on the NASA mission named NEO Surveillance Mission as part of the Follow-Up working group. His main contribution being in the development of a ranking algorithm needed for decision making related to NEOs follow-up strategies, along with the development of a medium-fidelity Near-Earth Objects simulator to be used to support and validate the ranking algorithm and the decision-making tool. His main research interest is the application of machine learning to several fields such as physics-informed solutions of space guidance problems and general optimal control problems, remote sensing for Earth systems characterization and space exploration.
Degrees
- PhD, Systems and Industrial Engineering, The University of Arizona (2022)
- MSc, Energy and Nuclear Engineering, University of Bologna (2016)
- BSc, Energy Engineering, University of Bologna (2013)
Publications
Journal Articles
-
De Florio, M., Schiassi, E., and Furfaro, R., Physics-informed neural networks and functional interpolation for stiff chemical kinetics, Chaos 32, 063107 (2022) https://doi.org/10.1063/5.0086649
- De Florio, M., Schiassi, E., Ganapol, B.D. et al. Physics-Informed Neural Networks for rarefied-gas dynamics: Poiseuille flow in the BGK approximation. Z. Angew. Math. Phys. 73, 126 (2022). https://doi.org/10.1007/s00033-022-01767-z
- Schiassi, E., De Florio, M., Ganapol, B.D., Picca, P. and Furfaro, R., 2021. Physics-informed neural networks for the point kinetics equations for nuclear reactor dynamics. Annals of Nuclear Energy, p. 108833. DOI: https://doi.org/10.1016/j.anucene.2021.108833
- De Florio, M., Schiassi, E., D’Ambrosio, A., Mortari, D. and Furfaro, R., 2021. Theory of Functional Connections Applied to Linear ODEs Subject to Integral Constraints and Linear Ordinary Integro-Differential Equations. Mathematics, 26(3), p.65. DOI: https://doi.org/10.3390/mca26030065
- Schiassi, E., De Florio, M., D’Ambrosio, A., Mortari, D. and Furfaro, R., 2021. Physics-Informed Neural Networks and Functional Interpolation for Data-Driven Parameters Discovery of Epidemiological Compartmental Models. Mathematics, 9(17), p.2069. DOI: https://doi.org/10.3390/math9172069
- E. Schiassi, R. Furfaro, C. Leake, M. De Florio, H. Johnston, D. Mortari, Extreme Theory of Functional Connections: A Fast Physics-Informed Neural Network Method for Solving Ordinary and Partial Differential Equations, Neurocomputing (2021). DOI: https://doi.org/10.1016/j.neucom.2021.06.015
- De Florio, M., Schiassi, E., Ganapol, B.D., Furfaro, R., "Physics-informed neural networks for rarefied-gas dynamics: Thermal creep flow in the Bhatnagar–Gross–Krook approximation", Physics of Fluids 33, 047110 (2021). DOI: https://doi.org/10.1063/5.0046181
- Drozd, K., Furfaro, R., Schiassi, E., Johnston, H., & Mortari, D. (2021). Energy-optimal trajectory problems in relative motion solved via Theory of Functional Connections. Acta Astronautica.
- De Florio, M., Schiassi, E., Furfaro, R., Ganapol, B.D., Mostacci, D. (2020). Solutions of Chandrasekhar’s Basic Problem in Radiative Transfer via Theory of Functional Connections. Journal of Quantitative Spectroscopy & Radiative Transfer. DOI: https://doi.org/10.1016/j.jqsrt.2020.107384
- Johnston, H., Schiassi, E., Furfaro, R., & Mortari, D. (2020). Fuel-Efficient Powered Descent Guidance on Large Planetary Bodies via Theory of Functional Connections. The journal of the astronautical sciences. PDF, DOI: 10.1007/s40295-020-00228-x
- Watson, C. S., Kargel, J. S., Shugar, D. H., Haritashya, U. K., Schiassi, E., & Furfaro, R. (2020). Mass loss from calving in Himalayan proglacial lakes. Frontiers in Earth Science, 7. DOI: http://dx.doi.org/10.3389/feart.2019.00342
- Bishop, M. P., Young, B. W., Colby, J. D., Furfaro, R., Schiassi, E., & Chi, Z. (2019). Theoretical Evaluation of Anisotropic Reflectance Correction Approaches for Addressing Multi-Scale Topographic Effects on the Radiation-Transfer Cascade in Mountain Environments. Remote Sensing, 11(23), 2728. DOI: http://dx.doi.org/10.3390/rs11232728
- Schiassi, E., Furfaro, R., Kargel, J. S., Watson, C. S., Shugar, D. H., & Haritashya, U. K. (2019). GLAM Bio-Lith RT: A Tool for Remote Sensing Reflectance Simulation and Water Components Concentration Retrieval in Glacial Lakes. Frontiers in Earth Science, 7. DOI: http://dx.doi.org/10.3389/feart.2019.00267
- Schiassi, E., Furfaro, R., & Mostacci, D. (2016). Bayesian inversion of coupled radiative and heat transfer models for asteroid regoliths and lakes. Radiation Effects and Defects in Solids, 171(9-10), 736-745. DOI: http://dx.doi.org/10.1080/10420150.2016.1253091
Conference Papers
- Furfaro, R., Scorsoglio, A., Schiassi, E., Physics-Informed Neural Networks for Hamilton Jacobi Bellman Equations for a class for optimal control problems. AAS 21-319, 31st AAS/AIAA Space Flight Mechanics Meeting. PDF
- Schiassi, E., D'Ambrosio, A., Johnston, H., De Florio, M., Furfaro, R., Curti, F., Mortari, D., Physics-Informed Extreme Theory of Functional Connections Applied to Optimal Orbit Transfer. AAS 20-524, AAS/AIAA Astrodynamics Specialist Conference, Lake Tahoe, CA, August 9-13, 2020. PDF
- D'Ambrosio, A., Schiassi, E., Curti, F., Furfaro, R., Physics-Informed Neural Networks Applied to a Series of Constrained Space Guidance Problems. AAS 21-259, 31st AAS/AIAA Space Flight Mechanics Meeting. PDF, Presentation PDF
- Schiassi, E., D'Ambrosio, A., Scorsoglio, A., Furfaro, R., Curti, F., Class of Optimal Space Guidance Problems solved via Indirect Methods and Physics-Informed Neural Networks. AAS 21-258, 31st AAS/AIAA Space Flight Mechanics Meeting. PDF, Presentation PDF
- Schiassi, E., D'Ambrosio, A., Furfaro, R., Curti, F., Physics-Informed Neural Networks for Optimal Intercept Problem. IAA-AAS-SciTech2020-76, 3-rd IAA-AAS SciTech Forum on Space Flight Mechanics and Space Structures. PDF, Presentation PDF
- Schiassi, E., D'Ambrosio, A., Johnston, H., Furfaro, R., Curti, F., Mortari, D., Complete Energy Optimal Landing on Small and Large Planetary Bodies via Theory of Functional Connections. AAS 20-557, AAS/AIAA Astrodynamics Specialist Conference, Lake Tahoe, CA, August 9-13, 2020. PDF
- Schiassi E., Furfaro R., Johnston H., & Mortari D. (2019). Fuel-efficient Powered Descent Guidance on Planetary Bodies via Theory of Functional Connection 1: Solution of the Equations of Motion, AAS 19-718, AAS/AIAA Astrodynamics Specialist Conference, Portland, ME. PDF
Preprints
- Schiassi, E., D'Ambrosio, A., De Florio, M., Furfaro, R., Curti, F. (2020). Physics-Informed Extreme Theory of Functional Connections Applied to Data-Driven Parameters Discovery of Epidemiological Compartmental Models. arXiv:2008.05554v1. PDF
- Schiassi, E., Leake, C., De Florio, M., Johnston, H., Furfaro, R., & Mortari, D. (2020). Extreme Theory of Functional Connections: A Physics-Informed Neural Network Method for Solving Parametric Differential Equations. arXiv :2005.10632. PDF
Conference Presentations
- Los Alamos - Arizona days, Online Workshop, 19-19 May 2020. Physics-Informed Solutions of Radiative Transfer Problems. Presentation.pptx
- AAS Conference, Portland, Maine (USA), 11-15 August 2019. Fuel-efficient Powered Descent Guidance on Planetary Bodies via Theory of Functional Connections. Presentation PDF
- HiMAT meeting, Seattle, Washington (USA), 20-22 March 2018. Glacial Lake Assisted Melting (GLAM). Presentation PDF